3 min
#Expert Q&A: NJIT’s David Bader on AI, Data Science, Quantum Computing
Artificial intelligence, data science and the emerging field of quantum computing areamong the hottest research topics in computing today. David Bader, a distinguishedprofessor at New Jersey Institute of Technology’s Ying Wu College of Computing andthe director of the university’s Institute for Data Science, offers his take on each.The Computer History Museum has recognized you for developing a Linuxsupercomputer using commodity hardware. Was that a life-shaping lesson?It was a venture into the unknown, leveraging the emerging potential of Linux and commodity hardware to build something that was both accessible and powerful. This experience taught me the importance of embracing risk and the value of resilience. There were technical hurdles, skepticism from peers and the daunting task of venturing beyond established norms. Also, the Linux supercomputer project was not just about the technology. It was about building a community around an idea.How do user-friendly AI systems like ChatGPT impact your work?It enriches the palette of methodologies and technologies at our disposal, enabling us to tackle more ambitious projects with greater efficiency and creativity. By integrating these AI systems into our research and educational programs, we're not just enhancing our ability to process and analyze data. We're also empowering students and researchers with the means to innovate and explore new horizons in data science without being hindered by the technical complexities that once acted as barriers.Some information workers fear that AI will make their careers obsolete. But tech progress can’t be stopped, so how should people adapt?By embracing these technologies, learning to work alongside them and leveraging their capabilities to enhance our own skill sets and productivity. Also, it's important to focus on the uniquely human skills that AI cannot replicate, such as creativity, emotional intelligence and critical thinking. By honing these abilities, workers can ensure they remain irreplaceable components of the workforce, capable of tasks that require a human touch — from complex decision-making to empathetic interactions with customers or clients.What should non-programmers learn about AI?It’s important to be aware of how AI decisions are made, the potential biases in AI systems and the ethical considerations of AI use. Additionally, developing data literacy is crucial, as it enables individuals to evaluate AI outputs and understand the importance of data quality and biases. A basic grasp of AI and machine learning concepts — even without programming skills — can demystify AI technologies and reveal their potential applications. Staying informed about AI advancements across various sectors can also inspire innovative ideas and foster interdisciplinary collaborations.There’s a sci-fi plot where computers get so smart that people lose control. The new class of user-friendly AI is making people excited but also nervous. Should we be afraid?While it’s natural to harbor concerns about the rapid progression of AI, allowing fear to dominate the discourse would be a disservice to the potential benefits these technologies can offer. Instead, this moment calls for proactive engagement with AI and an investment in understanding its inner workings, limitations and the ethical dilemmas it presents. By advocating for responsible AI development, emphasizing education and promoting transparency, we can foster an environment where AI serves as a tool for societal advancement. This approach ensures that we remain at the helm of AI's trajectory, steering it toward outcomes that uplift humanity rather than scenarios that fuel dystopian fears.What other emerging technologies excite you in their potential to transformcomputing?Quantum computing. This technology, with its potential to solve complex problems exponentially faster than classical computers, could revolutionize fields ranging from cryptography to drug discovery, climate modeling and beyond. Quantum computing's promise to tackle challenges currently beyond our reach, due to its fundamentally different approach to processing information, represents a leap forward in our computational capabilities. Its convergence with AI could lead to unprecedented advancements, making this era an incredibly thrilling time to be at the forefront of computing and data science.Looking to know more? We can help.David Bader is available to discuss AI, quantum computing and data science withmedia. Simply click on his icon to arrange an interview.
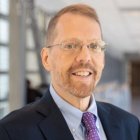