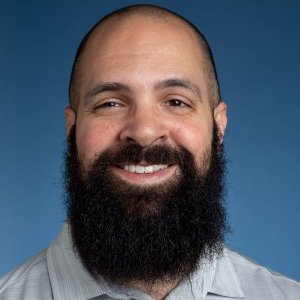
Andrew Forney
Associate Professor of Computer Science
Biography
Phone: 310.338.3077
Email: andrew.forney@lmu.edu
Office: Doolan 201A
Professor Andrew Forney is a third-generation Lion who completed his B.S. in Computer Science and Psychology at Loyola Marymount University and his M.S. and Ph.D. in Computer Science at UCLA. His research bridges the disciplines of cognitive psychology, artificial intelligence (AI), and experimental design, with an emphasis in causal inference. He is interested in the mechanics of counterfactual reasoning and its applications to human and machine learning, reinforcement, and decision-making for crafting increasingly dynamic and metacognitive agents. His work has been published and presented in the flagship AI conferences (NeurIPS, ICML, AAAI) and Journal of Causal Inference. Professor Forney’s other interests include pedagogical methods for inclusive and engaging undergraduate STEM experiences, and the entanglement of increasingly advanced AI in society. He leads the Applied Cognitive Technologies (ACT) Laboratory at LMU, which provides undergraduates with research and industry experience using state-of-the-art techniques in AI applied to real world problems.
Education
University of California, Los Angeles
Ph.D.
Computer Science
2018
University of California, Los Angeles
M.S.
Computer Science
2015
Loyola Marymount University
B.S.
Computer Science
2012
Areas of Expertise
Industry Expertise
Links
Media Appearances
Lions Develop App to Exonerate the Innocent
LMU print
2021-05-20
An expose on Briefcase, one of the ACT Lab's flagship projects using AI to help lawyers at LMU's Project for the Innocent to exonerate the wrongfully incarcerated.
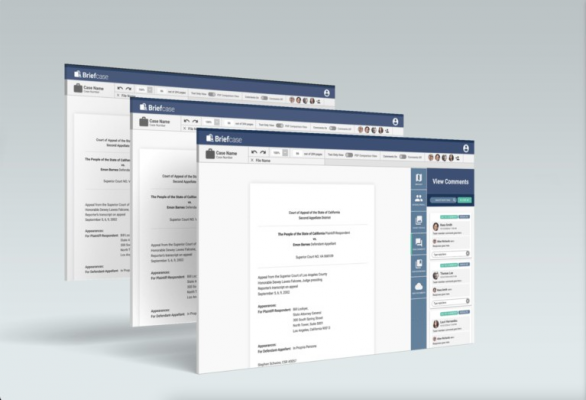
LMU Startup Demystifies the Arcane Legislative Process
LMU print
2019-12-05
Highlighting the efforts of LMU Alumni and ACT Lab graduates Patrick Utz and Mo Hayat in creating a multi-million dollar startup from their senior project. See https://www.abstract.us/ for recent developments.
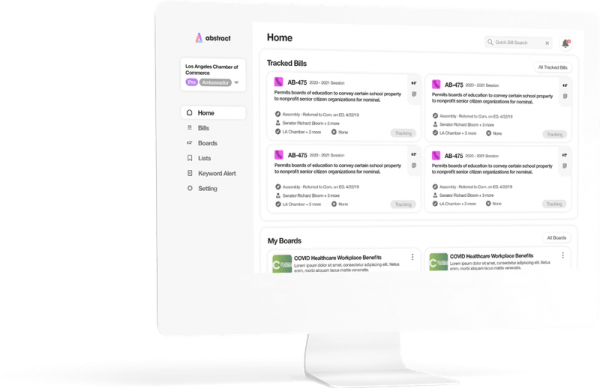
Articles
Causal Inference in AI Education: A Primer
Journal of Causal InferenceA. Forney, S. Mueller
2022-09-01
The study of causal inference has seen recent momentum in machine learning and artificial intelligence (AI), particularly in the domains of transfer learning, reinforcement learning, automated diagnostics, and explainability (among others). Yet, despite its increasing application to address many of the boundaries in modern AI, causal topics remain absent in most AI curricula. This work seeks to bridge this gap by providing classroom-ready introductions that integrate into traditional topics in AI, suggests intuitive graphical tools for application to both new and traditional lessons in probabilistic and causal reasoning, and presents avenues for instructors to impress the merit of climbing the “causal hierarchy” to address problems at the levels of associational, interventional, and counterfactual inference. Lastly, this study shares anecdotal instructor experiences, successes, and challenges integrating these lessons at multiple levels of education.
Exploiting Causal Structure for Transportability in Online, Multi-Agent Environments
Proceedings of the 21st International Conference on Autonomous Agents and Multiagent SystemsA. Browne, A. Forney
2022-09-05
Autonomous agents may encounter the transportability problem when they suffer performance deficits from training in an environment that differs in key respects from that in which they are deployed. Although a causal treatment of transportability has been studied in the data sciences, the present work expands its utility into online, multi-agent, reinforcement learning systems in which agents are capable of both experimenting within their own environments and observing the choices of agents in separate, potentially different ones. In order to accelerate learning, agents in these Multi-agent Transport (MAT) problems face the unique challenge of determining which agents are acting in similar environments, and if so, how to incorporate these observations into their policy. We propose and compare several agent policies that exploit local similarities between environments using causal selection diagrams, demonstrating that optimal policies are learned more quickly than in baseline agents that do not. Simulation results support the efficacy of these new agents in a novel variant of the Multi-Armed Bandit problem with MAT environments.
Counterfactual randomization: rescuing experimental studies from obscured confounding
Proceedings of the AAAI Conference on Artificial Intelligence 33 (01), 2454-2461A. Forney, E. Bareinboim
2019-07-17
Randomized clinical trials (RCTs) like those conducted by the FDA provide medical practitioners with average effects of treatments, and are generally more desirable than observational studies due to their control of unobserved confounders (UCs), viz., latent factors that influence both treatment and recovery. However, recent results from causal inference have shown that randomization results in a subsequent loss of information about the UCs, which may impede treatment efficacy if left uncontrolled in practice (Bareinboim, Forney, and Pearl 2015). Our paper presents a novel experimental design that can be noninvasively layered atop past and future RCTs to not only expose the presence of UCs in a system, but also reveal patient-and practitioner-specific treatment effects in order to improve decision-making. Applications are given to personalized medicine, second opinions in diagnosis, and employing offline results in online recommender systems.
Counterfactual data-fusion for online reinforcement learners
Proceedings of the 34th International Conference on Machine Learning-Volume 70A Forney, J Pearl, E Bareinboim
2017-08-06
Abstract
The Multi-Armed Bandit problem with Unobserved Confounders (MABUC) considers decision-making settings where unmeasured variables can influence both the agent's decisions and received rewards (Bareinboim et al., 2015). Recent findings showed that unobserved confounders (UCs) pose a unique challenge to algorithms based on standard randomization (i.e., experimental data); if UCs are naively averaged out, these algorithms behave sub-optimally, possibly incurring infinite regret. In this paper, we show how counterfactual-based decision-making circumvents these problems and leads to a coherent fusion of observational and experimental data. We then demonstrate this new strategy in an enhanced Thompson Sampling bandit player, and support our findings' efficacy with extensive simulations.
Bandits with unobserved confounders: A causal approach
Neural Information Processing Systems Conference (NeurIPS)E. Bareinboim, A. Forney, J. Pearl
2015-12-07
Abstract
The Multi-Armed Bandit problem constitutes an archetypal setting for sequential decision-making, permeating multiple domains including engineering, business, and medicine. One of the hallmarks of a bandit setting is the agent's capacity to explore its environment through active intervention, which contrasts with the ability to collect passive data by estimating associational relationships between actions and payouts. The existence of unobserved confounders, namely unmeasured variables affecting both the action and the outcome variables, implies that these two data-collection modes will in general not coincide. In this paper, we show that formalizing this distinction has conceptual and algorithmic implications to the bandit setting. The current generation of bandit algorithms implicitly try to maximize rewards based on estimation of the experimental distribution, which we show is not always the best strategy to pursue. Indeed, to achieve low regret in certain realistic classes of bandit problems (namely, in the face of unobserved confounders), both experimental and observational quantities are required by the rational agent. After this realization, we propose an optimization metric (employing both experimental and observational distributions) that bandit agents should pursue, and illustrate its benefits over traditional algorithms.
Can avatars pass the Turning test? Intelligent agent perception in a 3D virtual environment
International Journal of Human-Computer StudiesRL Gilbert, A Forney
2015-01-01
Abstract
The current study involved the first natural language, modified Turing Test in a 3D virtual environment. One hundred participants were given an avatar-guided tour of a virtual clothing store housed in the 3D world of Second Life. In half of the cases, a human research assistant controlled the avatar-guide; in the other half, the avatar-guide was a visually indistinguishable virtual agent or “bot” that employed a chat engine called Discourse, a more robust variant of Artificial Intelligence Markup Language (AIML). Both participants and the human research assistant were blind to variations in the controlling agency of the guide. The results indicated that 78% of participants in the artificial intelligence condition incorrectly judged the bot to be human, significantly exceeding the 50% rate that one would expect by chance alone that is used as the criterion for passage of a modified Turing Test. An analysis of participants׳ decision-making criteria revealed that agency judgments were impacted by both the quality of the AI engine and a number of psychological and contextual factors, including the naivety of participants regarding the possible presence of an intelligent agent, the duration of the trial period, the specificity and structure of the test situation, and the anthropomorphic form and movements of the agent. Thus, passage of the Turing Test is best viewed not as the sole product of advances in artificial intelligence or the operation of psychological and contextual variables, but as a complex process of human–computer interaction.
The distributed self: Virtual worlds and the future of human identity (pp. 22-36)
Book: The Immersive InternetR Gilbert, A Forney
2013-06-01
Abstract
This theoretical work develops two central premises. The first is that human identity, defined as a person’s conception and expression of his or her individuality, is not fixed and immutable but changes in response to revolutionary developments in culture and technology. The second is that we are currently in the early phases of one of these profound techno-cultural transitions: the rise of 3D virtual worlds and the formation of a ubiquitous, photorealistic, seamlessly integrated, and massively scaled metaverse (Dionisio et al., in press) that will dramatically reshape our conception and experience of the self.
A Framework for Empirical Counterfactuals, or for All Intents, a Purpose
ProQuest: UCLA dissertationA Forney
2018-01-01
Abstract
Unobserved confounders (UCs) are factors in a system that affect a treatment and its outcome, but whose states are unknown. When left uncontrolled, UCs present a major obstacle to inferring causal relations from statistical data, which can impede policy making and machine learning. Control of UCs has traditionally been accomplished by randomizing treatments, thus severing any causal influence of the UCs to the treatment assignment, and averaging their effects on the outcome in each randomized group. Although such interventional data can be used to appropriately inform population-level decisions, unit-level decisions are best informed by counterfactual quantities that provide information about the UCs relating to each unit. That said, arbitrary counterfactual computation can be performed in only certain scenarios, or in possession of a fully-specified causal model that requires knowledge of the distribution over UC states.
The Identity Mapping Project: Demographic differences in patterns of distributed identity
Annual Review of Cybertherapy and Telemedicine 2015: Virtual Reality in Healthcare: Medical Simulation and Experiential InterfaceRL Gilberta, JSN Dionisiob, A Forney, P Dorinb
2016-01-28
Abstract
The advent of cloud computing and a multi-platform digital environment is giving rise to a new phase of human identity called15 “The Distributed Self.” In this conception, aspects of the self are distributed into a variety of 2D and 3D digital personas with the capacity to reflect any number of combinations of now malleable personality traits. In this way, the source of human identity remains internal and embodied, but the expression or enactment of the self becomes increasingly external, disembodied, and distributed on demand. The Identity Mapping Project (IMP) is an interdisciplinary collaboration between psychology and computer Science designed to empirically investigate the development of distributed forms of identity. Methodologically, it collects a large database of “identity maps”--computerized graphical representations of how active someone is online and how their identity is expressed and distributed across 7 core digital domains: email, blogs/personal websites, social networks, online forums, online dating sites, character based digital games, and virtual worlds. The current paper reports on gender and age differences in online identity based on an initial database of distributed identity profiles.